
Neural Network processor boosts performance of radar, lidar, vision applications
One of the most widespread approaches to run AI systems is making use of Graphics Processor Units (GPUs). Their advantage: Standard graphics controllers for everyday computers typically contain many GPUs running in parallel. With the right algorithms, such graphic devices can be repurposed to AI engines that offer considerable performance. This is one of the recipes of success for market players like Nvidia. But not everyone believes that this approach is really the best one. “This approach requires very high-end GPUs that in turn consume vast amounts of energy,” comments Pulin Desai, product marketing director for the Tensilica Vision DSP product line. According to Desai, the GPU approach might be suitable for high-end server farms in environments where power is not critical. “In the car, you have to deal with embedded systems where space and power are limited.”
With the Tensilica Vision C5 DSP, Cadence suggests a different way. The C5 offers highly concentrated computing power – it offers a performance of 1 TeraMAC (Multiply-Accumulate computing steps) at the area of less than one square millimeter. This high performance is owed to its VLIW vector processing instruction set with 128-way, 8-bit or 64-way, 16-bit single-instruction multiple data (SIMD) execution. The device is optimized for vision, radar, lidar and sensor fusion applications with high availability requirements. And, important, it is architected for multi-processor designs – after all, state-of-the art ADAS, computer vision and other sensor information processing applications in vehicles are increasingly based on complex, heterogeneous multiprocessor designs.

of the Vision C5 performance
The power efficiency of the C5 is about one order of magnitude better than that of comparable GPU-based solutions, Cadence claims. It also offers an edge over neural network hardware accelerators: Since it is reprogrammable (in contrast to specialized, hardware-based accelerators) it is more flexible and future-proof. And since its functionality is implemented entirely in software with the development tools being widely available, the development is much easier, says Desai. Neural Network libraries will be available once the final version of the C5 will hit the markets. Besides automotive applications which certainly will represent the largest market, Cadence also plans to sell it to manufacturers of drones and security systems or any other kind of applications where neural-network based recognition algorithms are used to perform object detection and recognition.
Related articles:
Cadence’s Tensilica Fusion G3 DSP features efficient C programming
Dream Chip shows ADAS processor on FDSOI
Embedded ADAS Algorithm Optimization with High-Performance DSP IP and CV Software Library
Renesas tackles autonomous driving with scalable platform
Image signal processor makes cars see
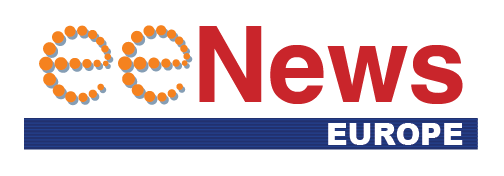